Computational Sustainability Seminar Series
Unless otherwise noted, seminars are held Fridays at 2:00 Eastern time in 5160 Upson Hall at Cornell, and online participation is available via WebEx. Please see the WebEx instructions with connection information listed under each seminar, or contact Megan McDonald at mm423@cornell.edu or Rich Bernstein at rab38@cornell.edu for further details.
Date/Time | Speaker | Title |
---|---|---|
Friday, September 21, 2012 at 2:00pm EDT | Stefano Ermon, Cornell University | Learning Policies For Battery Usage Optimization in Electric Vehicles |
Friday, October 26, 2012 at 2:30pm EDT | Marcelo Finger, University of Sao Paulo | Probabilistic Satisfiability: Algorithms and Phase transition |
Friday, November 2, 2012 at 2:00pm EdT | Shadi Atallah, Cornell University | An Agent-Based Model of Plant Disease Diffusion and Control: Grapevine Leafroll Disease |
Friday, November 30, 2012 at 2:00pm EST | Forrest Briggs, Oregon State University | Instance annotation for multi-instance multi-label learning, with applications to object recognition in images and bird song |
Friday, February 1, 2013 at 2:00pm EST | Eduardo Cotilla-Sanchez, Oregon State University | Multi-objective Partitioning of Electrical Power Networks |
Friday, March 8, 2013 at 2:00pm EST | Yohan Lee, Oregon State University | Deploying Initial Attack Resources for Wildfire Suppression: Spatial Coordination, Budget Constraints, and Capacity Constraints |
Stefano Ermon, Cornell University
Friday, September 21, 2012 at 2:00pm EDT
In 5160 Upson Hall (and online via WebEx, additional directions may be found here, or contact Megan McDonald at mm423@cornell.edu or Rich Bernstein at rab38@cornell.edu for further details)
Title: Learning Policies For Battery Usage Optimization in Electric Vehicles
Abstract:
The high cost, limited capacity, and long recharge time of batteries pose a number of obstacles for the widespread adoption of electric vehicles. Multi-battery systems that combine a standard battery with supercapacitors are currently one of the most promising ways to increase battery lifespan and reduce operating costs. However, their performance crucially depends on how they are designed and operated. In this paper, we formalize the problem of optimizing real-time energy management of multi-battery systems as a stochastic planning problem, and we propose a novel solution based on a combination of optimization, machine learning and data-mining techniques. We evaluate the performance of our intelligent energy management system on various large datasets of commuter trips crowdsourced in the United States. We show that our policy significantly outperforms the leading algorithms that were previously proposed as part of an open algorithmic challenge.
Marcelo Finger, University of Sao Paulo and visiting academic, Cornell University
Friday, October 26, 2012 at 2:30pm EDT
In 5160 Upson Hall (this seminar will be local only)
Title: Probabilistic Satisfiability: Algorithms and Phase transition
Abstract:
In this talk, we motivate the problem and present algorithms for probabilistic satisfiability (PSAT),
an NP-complete problem, focusing on the presence and absence
of a phase transition phenomenon for each algorithm.
Our study starts by defining a PSAT normal form, on which all algorithms are based.
Several forms of reductions of PSAT to classical
propositional satisfiability (SAT) are proposed. Theoretical and practical limitations of each algorithm
are discussed. Some algorithms are shown to present a phase transition behavior. We show that variations
of these algorithms may lead to the partial occlusion of the phase transition phenomenon and discuss
the reasons for this change of practical behavior.
Shady Atallah, Cornell University
Friday, November 2, 2012 at 2:00pm EDT
In 5160 Upson Hall ((and online via WebEx, additional directions may be found here, or contact Megan McDonald at mm423@cornell.edu or Rich Bernstein at rab38@cornell.edu for further details)
Title: An Agent-Based Model of Plant Disease Diffusion and Control: Grapevine Leafroll Disease
Shady S. Atallah, Miguel I. Gómez, Jon M. Conrad, and Jan P. Nyrop
Abstract:
The grapevine leafroll disease (GLRD) threatens grape harvests in the United States and the
world. This viral disease reduces yield, delays fruit ripening, and affects wine quality. The
disease ecology is still under study and the spatial-dynamics of the spread process remains
poorly understood. Moreover, little is known about cost-efficient strategies to control the disease.
In an effort to address this gap in the literature, we model GLRD diffusion in a vineyard and
evaluate bioeconomic outcomes under alternative disease control strategies. We employ agent-
based modeling (ABM) tools and contribute to bioeconomic literature on agricultural disease
control in several ways. First, our model relaxes the assumption of agent homogeneity and
allows instead agents to be heterogeneous in age and infection states, thus in their economic
values. Second, we make the model inherently spatial-dynamic by combining the ABM with
a cellular automaton system. Third, we incorporate realism when modeling the spread process
by making the disease onset and its transmission stochastic. That is, initial infections follow
a random spatial distribution and stochastic agent interaction gives rise to Markov process-
type disease diffusion. Finally, we formulate novel control strategies consisting of roguing
and replacing infected grapevines based on their age and infection states. We evaluate these
strategies and identify those that perform best at extending the expected vineyard half-life and
at maximizing the vineyard expected net present values relative to the baseline of no control.
The model results underscore the ecological and economic tradeoffs implied by disease control
strategies based on age and infection states.
Forrest Briggs, Oregon State University
Friday, November 30, 2012 at 2:00pm EST
In 5160 Upson Hall (and online via WebEx, additional directions will be available soon, or contact Megan McDonald at mm423@cornell.edu or Rich Bernstein at rab38@cornell.edu for further details)
Title: Instance annotation for multi-instance multi-label learning, with applications to object recognition in images and bird song
Abstract:
In supervised learning problems concerning images and sounds, it is
often natural to decompose a document (bag) into a collection of parts
(instances), and to associate each instance with a feature vector. It
is also natural to associate documents with multiple labels, for
example describing all objects in an image, or all species in an audio
recording of bird song. This structure motivates the multi-instance
multi-label (MIML) framework, where the goal is to learn a classifier
from a dataset consisting of bags of instances paired with sets of
labels. In a MIML dataset, instances are not labeled directly; only
bags are labeled. Labeling at this course scale usually requires less
human effort than labeling instances. Prior work on MIML has focused
on predicting the label set for a previously unseen bag. We instead
consider the problem of predicting instance labels, while learning
only from data labeled at the bag level. This problem is called
instance annotation for MIML.
We propose a regularized rank-loss objective designed for instance annotation, which can be instantiated with different aggregation models that link bag-level loss with instance-level predictions. We consider aggregation models that can be factored as a linear function of one "support instance" per class, which are feature vectors summarizing a bag. Hence we name our proposed method rank-loss Support Instance Machines. We propose two optimization methods for the rank-loss objective, which is non-convex. One is a heuristic that alternates between updating support instances and solving a convex problem in which the support instances are treated as constant. The other is to apply the constrained concave-convex procedure (CCCP), which solves a similar convex problem in each step. We solve the convex problems using the Pegasos framework for primal sub-gradient descent, and prove that it finds an epsilon-suboptimal solution in runtime that is linear in the size of the dataset and O(1/epsilon). Additionally, we suggest a method of extending the linear learning algorithm to non-linear classification without increasing asymptotic runtime.
Experiments show that the proposed methods achieve better accuracy than recent methods based on other loss functions. The datasets in these experiments include field-collected recordings of bird song with multiple simultaneously vocalizing birds, and the Microsoft Research Cambridge and PASCAL VOC 2012 machine vision datasets.
Eduardo Cotilla-Sanchez, Oregon State University
Friday, February 1, 2013 at 2:00pm EST
In 5160 Upson Hall (and online via WebEx, additional directions will be available soon, or contact Megan McDonald at mm423@cornell.edu or Rich Bernstein at rab38@cornell.edu for further details)
Title: Multi-objective Partitioning of Electrical Power Networks
Abstract:
Identifying coherent sub-graphs in networks is important in many applications. In power systems, large systems are divided into areas and zones to aid in planning and control applications. But not every partitioning is equally good for all applications. In this talk we will discuss a hybrid method that combines a conventional graph partitioning algorithm with a genetic algorithm to partition a power network based on electrical distances, cluster sizes, the number of clusters, and cluster connectedness. Clusters produced by this method can be used to identify buses with dynamically coherent voltage angles, without the need for dynamic simulation. This also results in intra-zone transactions that have less impact on power flows outside of the zone, a property particularly useful for power system applications where ensuring deliverability is important, such as transmission planning or determination of synchronous reserve zones.
Yohan Lee, Oregon State University
Friday, March 8, 2013 at 2:00pm EST
In 5160 Upson Hall (and online via WebEx, additional directions will be available soon, or contact Megan McDonald at mm423@cornell.edu or Rich Bernstein at rab38@cornell.edu for further details)
Title: Deploying Initial Attack Resources for Wildfire Suppression: Spatial Coordination, Budget Constraints, and Capacity Constraints
Abstract:
We combine a scenario-based, standard-response optimization model with stochastic simulation to improve the efficiency of resource deployment for initial attack on wildland fires in three planning units in California. The optimization model minimizes the expected number of fires that do not receive a standard response—defined as the number of resources by type that must arrive at the fire within a specified time limit—subject to budget and station capacity constraints and uncertainty about the daily number and location of fires. We use the California Fire Economics Simulator to predict the number of fires not contained within initial attack modeling limits. Compared with the current deployment, the deployment obtained with optimization shifts resources from the planning unit with highest fire load to the planning unit with the highest standard response requirements leaving simulated containment success unchanged. This result suggests that, under the current budget and capacity constraints, a range of deployments may perform equally well in terms of fire containment. When constraints on firefighting budget and station capacity are relaxed, the optimization produces deployments with greater containment success, suggesting that fire suppression effectiveness will be negatively impacted by declining budgets and improved by consolidating existing resources into fewer stations.
Drop us an email if you'd like to join us in establishing the field of Computational Sustainability.
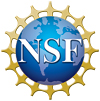
Sponsored by the National Science Foundation