Master Class (Saturday, May 18, 2013)
Computational Sustainability: Optimization and Policy-Making
Chair
Barry O'Sullivan, University College Cork, Ireland
Sponsor
Institute for Computational Sustainability, Cornell University
Schedule | |
---|---|
7:50am | Bus Pickup from Holiday Inn, Mount Kisco |
08:30 - 9:00am | Introduction: Optimization and Policy-Making in Computational Sustainability [slides] Barry O'Sullivan, University College Cork |
09:00 - 10:30am | Class 1: Smarter Planet Initiatives Jayant Kalagnanam, IBM Research Abstract |
10:30 - 11:00am | Morning Break (Mezzanine) |
11:00 - 12:30pm | Class 2: Models and Algorithms for Energy Markets with High Penetrations of Renewables [slides] Warren B. Powell, Princeton University Abstract |
12:30 - 2:00pm | Lunch (Cafeteria) |
2:00 - 3:30pm | Class 3: Solving Stochastic Optimization Problems with Bounded Risk Through Risk Allocation [slides] Brian Williams, Massachusetts Institute of Technology Abstract |
3:30 - 4:00pm | Afternoon Break (Mezzanine) |
4:00 - 5:30pm | Class 4: Zombie Optimization or How I Learned to Love Decomposition [slides] J. Christopher Beck, University of Toronto Abstract |
5:30 - 6:00pm | Wrap-up discussion |
Smarter Planet Initiatives
Jayant Kalagnanam
Strategic Growth Initiatives, Business Analytics & Math Sciences
IBM Thomas J. Watson Research Center, Yorktown Heights, NY USA
Abstract: In this session I will provide an overview of IBM's thrust with Smarter Planet and related projects. This initiative started in IBM several years ago with a belief that information technology can play a central role in harnessing the data from an instrumented, interconnected world and bring intelligence to improve decision making at all levels of society to build a more sustainable planet. Several emerging trends such as the proliferation of interconnected smart devices point to an increased availability of information to estimate the state of the human and earth systems. This coupled with novel decision making approaches can significantly change the way we manage the emerging instrumented planet. In this session I will discuss several problems and projects that emerge from two areas of applications: (i) Smart Grids for Electricity production and consumption, and (ii) Predictive Environmental Analysis Systems.
Models and Algorithms for Energy Markets with High Penetrations of Renewables [slides]
Warren B. Powell
Director, Princeton Laboratory for Energy Systems Analysis
Department of Operations Research and Financial Engineering
Princeton University
Abstract: Energy markets are particularly challenging to model when we have to grapple with the uncertainties introduced by high penetrations of renewables. The technology of deterministic models is well understood, but the introduction of significant levels of uncertainty requires that we properly capture the flow of information and the different mechanisms for adapting to it. Energy markets are nested: day-ahead plans have to anticipate that hour-ahead planning can respond to information at different times of the day. Demand response markets can operate with zero to several hours advance notice. Decisions in the day ahead market ripple through the intermediate and real-time markets, introducing a modeling challenge that is not handled using familiar modeling frameworks such as stochastic programming, dynamic programming or simulation. This tutorial will introduce students to the fundamental tools for modeling stochastic, dynamic problems, which will then be demonstrated in the context of capturing the dynamics of energy markets. We separate the process of modeling from the design of policies for making decisions. We summarize the four classes of policies, and then demonstrate how hybrids can be used to handle the nesting of day-ahead and hour-ahead markets.
Solving Stochastic Optimization Problems with Bounded Risk Through Risk Allocation [slides]
Brian C. Williams
MIT CSAIL
Abstract: The concepts of optimality, uncertainty and risk go hand in hand with sustainability. In the arena of energy management, grids of renewables must dispatch energy while managing high uncertainty in supply and demand, and while bounding the risk of not meeting demand. Energy efficient homes must balance energy saving against the risk of user discomfort, given that occupant behavior, as well as supply is uncertain. Drivers of plug-in electric vehicles must over come the range anxiety of being stranded in a traffic jam. In the arena of environmental monitoring, observing systems comprised of autonomous vehicles must balance information gain and level of operator involvement against the risk of vehicle loss. Essential to many of these decision problems is that their solutions meet an upper bound on the risk of failure. This can be addressed by framing these decision problems as stochastic constraint optimization problems in which bounds on risk are specified through global chance constraints. A second essential feature is that these problems be solved on line, in order to adapt to changes in the environment. Solving chance-constrained problems online is notoriously difficult. For example, sampling methods, while broadly applicable, incur an enormous computational cost. In this class we explore an emerging family of solution methods centered on the metaphor of risk allocation. In these methods a global risk bound is viewed as a resource that can be “spent” in order to decide how much risk to take of violating each individual constraint. Given a risk allocation, the stochastic problem can be reformulated to a deterministic problem that often introduces only a smallamount of conservatism. The optimal risk allocation is then one that maximizes expected utility while satisfying the global risk bound. In the class we will explore the solution to convex and non-convex problems using closed form and iterative approaches to risk allocation. These methods will be presented in the context of energy management and observing applications. Joint work with Masahiro Ono (Caltech JPL) and Lars Blackmore (Space X).
Zombie Optimization or How I Learned to Love Decomposition [slides]
J. Christopher Beck
Department of Mechanical & Industrial Engineering
University of Toronto
Abstract: Decomposing a problem into manageable pieces, where each piece can be modeled and solved using existing optimization tools, is one of the key ways in which we address large problems. Many of the problem classes we spend our time studying in optimization are really part of a much larger system. For example, think of the role of scheduling a factory within the larger supply chain system that includes other factories, suppliers, inventory management, logistics, process planning, product design, organizational design, personnel training, strategic management, etc. This larger systems view is important in computational sustainability as the problems we model and solve lie in the context of a much larger eco-system.
In this talk, I will present work on a particular pattern of problem decomposition that has been developed over the last decade and is best represented within the CPAIOR community as logic-based Benders decomposition. This is a powerful pattern with different instantiations in AI and OR. I will present a number of these instantiations, illustrating with work, where possible, on problems of computational sustainability. Time-permitting, I will also make connections to traditional modeling and search in constraint programming and provide some guesses as to where the research might be going.

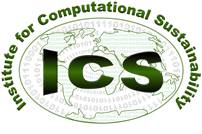


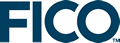





